일 | 월 | 화 | 수 | 목 | 금 | 토 |
---|---|---|---|---|---|---|
1 | 2 | 3 | 4 | 5 | ||
6 | 7 | 8 | 9 | 10 | 11 | 12 |
13 | 14 | 15 | 16 | 17 | 18 | 19 |
20 | 21 | 22 | 23 | 24 | 25 | 26 |
27 | 28 | 29 | 30 |
- coco
- Realtime
- Transformer #classification #SOTA #Google
- CVPR
- hourglass
- DeepDoubleDescent #OpenAI #어려워
- POSE ESTIMATION
- IvanLaptev
- 정리용 #하나씩읽고있음 #많기도많네 #내가찾는바로그논문은어디에
- objectdetector
- vit
- centernet
- np-hard
- XiaomingLiu #PersonIdentification
- Manmohan #UnconstrainedComputerVision
- ICCV19 #Real-World #FaceRecognition
- cornernet #simple #다음은centernet #hourglass생명력이란
- Today
- Total
목록분류 전체보기 (16)
HyperML
- 회복 탄력성 (NVidia)[Jensen Huang] https://www.youtube.com/shorts/X6giT3YRT6U - 핵심가치 (Apple)[Steve Jobs] Think different https://www.youtube.com/watch?v=EWSA7Lykvt4 - 스트레스 (Amazon)[Jeff Bezos] https://www.youtube.com/shorts/WvNVRnAgFt0 - 핵심가치 (Amazon 4:10~) https://www.youtube.com/watch?v=l9X8X-Ixbo8&t=1230s
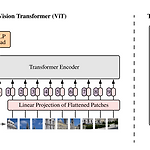
Transformer 는 본래 attention mechanisim 에 기반하여 language model의 학습을 위해 설계되었다. 간단한 구조와 적은 inductive bias 및 큰 weight capacity로 거대하게 모델을 만들고 거대한 데이터 학습에도 그 성능이 포화되지 않고, 언어모델의 self-supervised 학습 -> finetune 과정을 쉽게 수행함으로써 BERT, GPT와 같은 거대모델의 출현 및 다양한 task 활용을 이끌어 자연어 처리분야의 사실상 표준(de-facto standard)이 되었다. 이러한 transformer가 vision task에 적용될 것이라는 것은 누구나 예상할 수 있었고 많은 시도가 있었지만, ViT에 이르러서야 구글의 많은 데이터로 vision c..
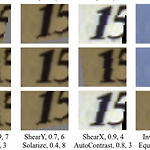
Introduction - Data augmentation은 전문가의 손길과 설계 policy를 위해서는 매뉴얼한 조작이 필요합니다. - 현재까지 소개되었던 학습가능한 augmentation policy 기법들은 정확도, 모델의 견고성과 성능을 높여주었습니다. - NAS(Neural Architecture Search)기반의 최적화 방법은 더 나은 예측 성능을 높였으나 복잡성과 엄청난 계산량 요구때문에 기피되었습니다. - 그래서 좀 더 효율적인 방식의 augmentation 함수의 탐색기법으로 AutoAugment(18.05, Google Brain), Fast AutoAugment(19.05 Kakao Brain) 같은 방식이 제안되었습니다. - 그럼에도 불구하고 ML 모델의 학습에 여전히 비용이 많이..
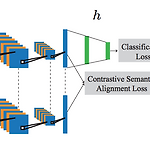
Domain adaptation이란 일반적으로 사람들은 imagenet이나 coco dataset으로 학습한 모델을 사용하여, 내가 하고자 하는 task에 적용을 해본다음 성능을 높이기 위해, transfer learning등의 단계로 넘어간다. imagenet과 같은 학습용 open dataset은 구하기 쉬우나, 세상 모든 task에 대응할 수는 없다. 이를테면 공사현장 cctv등을 감시하는 시스템을 개발한다고 하면, imagenet이나 coco person에서 학습한 object detector 모델을 그대로 사용하여 detection등을 수행할 경우, 처참한 결과를 보게 된다. 여기서 domain adaptation의 필요가 생겨났다. 이미 유사하게 학습한 모델을 적은 노력을 들여서 catastr..
Surveys: https://arxiv.org/pdf/1802.03601.pdf https://arxiv.org/pdf/1702.05374.pdf Awesome DA: https://porter.io/github.com/artix41/awesome-transfer-learning Porter.io Porter.io porter.io SDA(Supervised Domain Adaptation): CCSA: Unified Deep Supervised Domain Adaptation and Generalization (2017) FADA: Few-Shot Adversarial Domain Adaptation (2017) Augmented-Cyc: Augmented Cyclic Adversarial Learn..
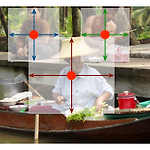
두 가지 종류의 CenterNet이 있다 하나는 본 논문 Objects as Points 나머지 하나는 CenterNet: Keypoint Triplets for Object Detection 둘다 arxiv.org 기준으로 19.04에 등재되었다. 지난번 소개한 CornerNet 과 같이 keypoint heatmap 방식으로 object detection을 수행하는 논문들이 늘고 있는데 본 논문도 그런 흐름위에 있다. Centernet의 특징은? - 별도의 anchorbox없이 object detection을 object의 중앙에 놓인 point의 heatmap으로 결정한다는 점 - 중앙 point의 feature값으로 detection뿐 아니라 object size, dimension, 3D ext..
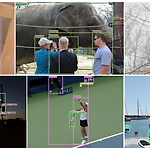
CornerNet은 1-stage 계열에서 새로운 방식으로 등장한 object detector이다. 일반적으로 box를 나타내는 top-left, bottom-right 정보를 바로 keypoint detection으로 찾는 방식인데, 마치 2d human pose estimation에서 heatmap을 가지고 joint의 keypoint를 찾는 것과 유사하다. 기존의 1-stage detector와 무엇이 다른가? 1. bounding box를 pair corner point로 찾고 각각의 포인트의 embedding을 통해 pair를 구성한다. 100k에 이르는 anchor box가 필요하지 않다 2. corner pooling : left-most, top-most 검색을 하고, max인 교차점을 구..
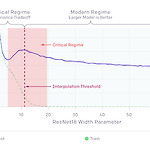
원문 : https://openai.com/blog/deep-double-descent/?fbclid=IwAR2kjb-SCR2wEWWlIKk3lnzVh9y_VYIInryB-DH7gBIcApi4xfdKRllnlx8 Deep Double Descent We show that the double descent phenomenon occurs in CNNs, ResNets, and transformers: performance first improves, then gets worse, and then improves again with increasing model size, data size, or training time. This effect is often avoided through careful op..
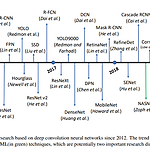
"Recent Advances in Deep Learning for Object Detection" 출처 : https://arxiv.org/pdf/1908.03673v1.pdf 제목 링크 출판연도 관련링크 FCOS: Fully Convolutional One-Stage Object Detection https://arxiv.org/pdf/1904.01355.pdf 19.04 https://github.com/tianzhi0549/FCOS CenterNet: Keypoint Triplets for Object Detection https://arxiv.org/pdf/1904.08189.pdf 19.04 https://github.com/xingyizhou/CenterNet NAS-FPN: Learning..